AI vs Traditional Data Science: Which One Delivers Better ROI?
- Date March 8, 2025
AI vs Traditional Data Science: Artificial intelligence involves machines where people can study data, learn things almost on their own, find all sorts of cool patterns, and make good decisions with help from humans, too. This flexibility lets it work well when it comes to automation and tackling complicated problems.
Meanwhile, conventional data science is about crunching numbers and extracting valuable information from everything, from neat spreadsheets to messy emails and articles. It does this by using fancy statistics and other techniques like mining data and using machine learning. Finding trends and making calls is based on the human brain. The problem is that these human brains are important and are extremely good at making decisions.
In a world where data dictates decisions, companies face a critical choice: how to measure the return on their technology investments. The right approach can maximize efficiency, reduce costs, and improve results. The purpose of this article is to analyze and compare ROI with traditional data science.
By understanding these differences, companies can make large investments based on data, which helps them to be extremely efficient, save money, and truly drive innovation.
Must Read: The Rise of AI in Data Science
Best Data Science Course in 2025
If you’re looking to build a strong career in data science, our EICTA, IIT Kanpur course is the perfect choice. This course is designed to help you master AI-driven automation as well as traditional data science techniques, giving you the best of both worlds. With industries relying more on data for decision-making, having the right skills is more important than ever. Learn from top experts, work on real-world projects, and get certified by IIT Kanpur—a credential that will make you stand out in the job market.
Professional Certification in AI Data Science 2025: Enroll Today!!
Advanced Certification Course in AI Data Science 2025: Enroll Today!!
Cost and Implementation
Cost is a significant factor when comparing AI and traditional data science. AI requires substantial investment in infrastructure, talent, and computing power, whereas traditional data science has a lower upfront cost but depends heavily on human expertise.
AI Implementation Costs and Complexity
Implementing AI involves high initial costs due to:
- Hardware and Infrastructure: AI models require GPUs, cloud computing, and scalable storage solutions.
- Data Preparation: To really train effectively, you’ve got to clean out and label big stacks of data first.
- Talent Acquisition: There’s a real gold rush going on for AI whizzes, engineers who work with data, and experts at making smart machines. They’re in such high demand that it’s costing companies a pretty penny just to hire these talents.
Must Read: How to Choose the Best AI Data Science Course in 2025
While automation through AI sure reduces expenses in the long run, upfront expenses can still be a real stumbling block for most companies.
Traditional Data Science Setup and Maintenance
While traditional data science has a lower hurdle to overcome, advanced data science is a bit trickier to break into, as:
- It uses structured datasets from relational databases.
- Relies on standard tools like Python, R, and SQL.
- Requires data analysts instead of AI specialists.
But keeping things manual and having to keep doing updates all the time is like building a system that keeps getting more and more costly to maintain over time.
Interested in E&ICT courses? Get a callback !
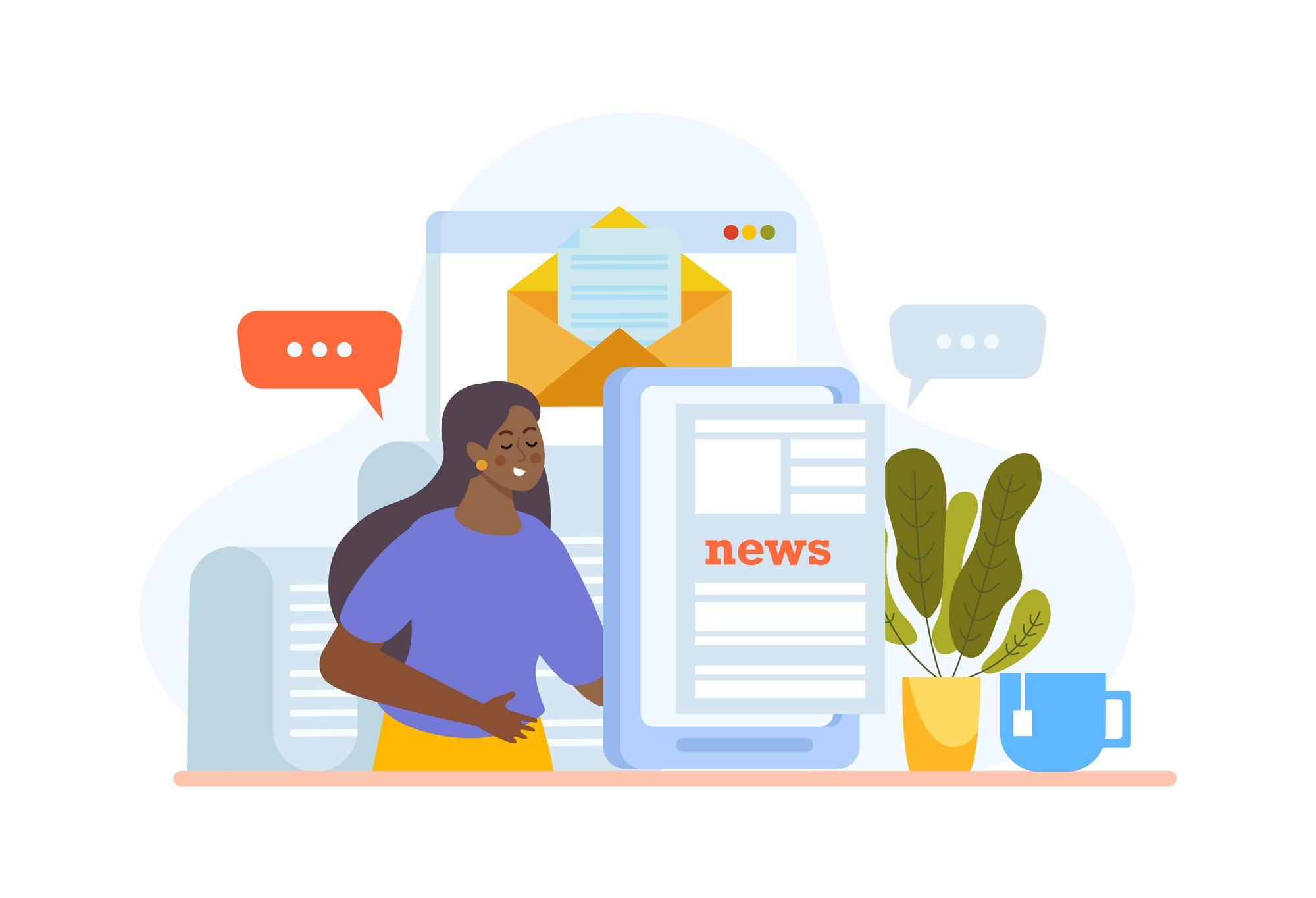
Performance and Efficiency
The effectiveness of AI and traditional data science depends on accuracy, automation, and adaptability. AI excels in handling vast, unstructured datasets, while traditional data science offers transparency and consistency.
AI's Accuracy, Automation & Adaptability
Unlike regular ways of thinking, AI learns new things dynamically and constantly improves without direct human tinkering. Automation lets business operations scale up smoothly and effectively, cutting costs and minimizing mistakes. AI-powered systems, such as recommendation engines and fraud detection models, continuously refine predictions, making them highly effective in evolving environments.
Must Read: Data Scientist vs Data Analyst
Traditional Data Science Reliability & Scalability
While automation has its place and merits, traditional methods have a way of just trusting less and sticking to proven basics. Models in stats and machine learning produce results that stand firm and whose reasoning can be understood easily.
Sure, traditional data science works more smoothly with predictable things compared to newer technologies like language learning models. It follows a clear, rule-based structure—if A happens, B follows. This method may lack the fluid adaptability of modern learning models, which refine themselves over time, but it offers something just as valuable: stability. With its structured logic, it delivers consistent results without the heavy computational demands of AI-driven systems. In an era of rapid innovation, sometimes reliability is its own kind of intelligence.
Scalability and Business Impact
Scalability plays a crucial role in determining ROI. AI is ideal for large-scale automation, while traditional data science remains valuable for structured data analysis.
AI for Large-Scale Automation and Real-Time Processing
AI-powered solutions benefit industries with high data volumes:
- Retail: Personalized recommendations increase sales.
- Healthcare: AI analyzes patient data for early diagnosis.
- Finance: Real-time fraud detection enhances security.
By scaling operations without additional manpower, AI offers a strong ROI for data-intensive industries.
Must Read: Data Scientist vs Data Engineer
Traditional Data Science in Structured Analysis
Traditional data science is well-suited for:
- Financial modeling, ensuring regulatory compliance.
- Market research, analyzing customer behavior trends.
- Risk assessment, evaluating structured datasets for decision-making.
While AI may not scale as fast, it also brings huge benefits like long-term stability and control and works great for businesses that need their data set up nicely.
Measuring ROI
To determine which approach delivers better ROI, companies must consider cost-benefit analysis and real-world case studies.
Cost Benefit Analysis
- AI: Higher initial costs but greater automation and efficiency.
- traditional data science: Lower costs but requires human intervention.
- Industry Impact: AI shines in fast-moving industries, Data Science works hard to make decisions using reliable data.
Must Read: Top Python Course
Case Studies
Daily Harvest, a meal delivery service, leveraged AI to enhance customer experience and streamline logistics with a small team. By implementing AI-driven personalized recommendations, automated customer support, and optimized packaging using weather-based calculations, the company reduced operational costs and improved customer satisfaction.
Must Read: How to Become Data Scientist with No Experience
Similarly, 3 Men Movers, a moving company from Texas, used AI to boost both the safety and efficiency of the drivers. Their AI-enabled distracted-driver detection system identified unsafe behaviors with 91% accuracy, reducing accidents by 4.5% in just three months. Additionally, AI-powered route optimization minimized liability and improved operational efficiency. Both firms have used AI in a big way to reduce costs, to keep folks much safer, and to improve all kinds of performance overall.
Conclusion
With that said, AI and traditional data science are both super helpful, but for different reasons. AI is great at coming up with super innovative solutions for demanding, nuanced problems that get people excited. Traditional data science, on the other hand, offers a counterbalance—an anchor in the storm. It brings structure, rigor, and a methodical approach to organizing vast quantities of information, uncovering patterns that might otherwise go unnoticed.
For businesses charting their long-term course, neither can be ignored. Decisions about where to invest, what strategies to pursue, and how to interpret market signals depend on understanding both the power of AI’s dynamism and the precision of data science.
Want to explore the nuances of AI and data science further? Check out our blogs!