AI vs Traditional Data Analytics: Why AI is the Game-Changer for 2025 Data Professionals
- Date May 12, 2025
In 2025, data is not the new oil—it’s the oxygen of innovation. Organizations everywhere rely on data-driven insights to optimize operations, predict trends, and compete with others. Traditional data analytics has been the backbone of decision-making for years now. But the situation is changing very fast. AI is no longer a future expectation—it’s actually transforming operational paradigms and redefining how industries operate at their core. For data professionals, this is not an upgrade—it’s a revolution.
Here‘s why AI vs traditional data analytics is the defining struggle of our time and how you can spearhead it.
Traditional Data Analytics: The Bedrock of the Past
Traditional data analysis has been the standard approach to deriving insights from structured data sets for many years. With applications such as Excel, and SQL, and Business Intelligence tools like Tableau, experts examine past data in order to respond to questions such as “What occurred?” or “Why did this occur?”. For instance, a company can utilize quarterly income statements to discover seasonal patterns or diagnose revenue decline.
Strengths of Traditional Methods
Clarity in Structured Data: Best suited for well-organized, numerical data.
User-Friendly Tools: Tools such as Power BI make visualization easy.
Established Practices: Well-known and relied upon in industries.
Limitations Holding Back Progress
But traditional analytics falls behind on current needs:
Manual Processes: Data cleaning and reporting construction waste are precious time.
Reactive Insights: Only concerned with the past and provides minimal insight into the future.
Organized Data Bias: Overlooks formats like free-form text, visual media, and multimedia content.
Scalability Challenges: Ineffectiveness in managing large or real-time data.
The AI Revolution: Revolutionizing Data Analytics
AI, driven by machine learning (ML) and deep learning, transforms data analysis into a different game. Unlike predefined rule-based algorithms, AI evolves from continuous learning, can adapt to new data trends, and makes independent decisions. From forecasting customer behaviour to automating intricate business processes, AI applications are numerous and expanding.
Why AI Beats Conventional Analytics
AI Outperforms traditional analytics with its flexibility, speed, depth of insights, and real-time responsiveness.
Automation: AI takes care of mundane tasks such as data preprocessing, allowing professionals to concentrate on strategy.
Predictive Power: Forecasts future trends (e.g., demand spikes, equipment failures).
Prescriptive Insights: Recommends actions (e.g., optimal pricing or inventory adjustments).
Unstructured Data Mastery: Analyzes text (via NLP), images (via computer vision), and sensor data.
Real-Time Processing: Delivers instant insights for industries like finance and healthcare.
Interested in E&ICT courses? Get a callback !
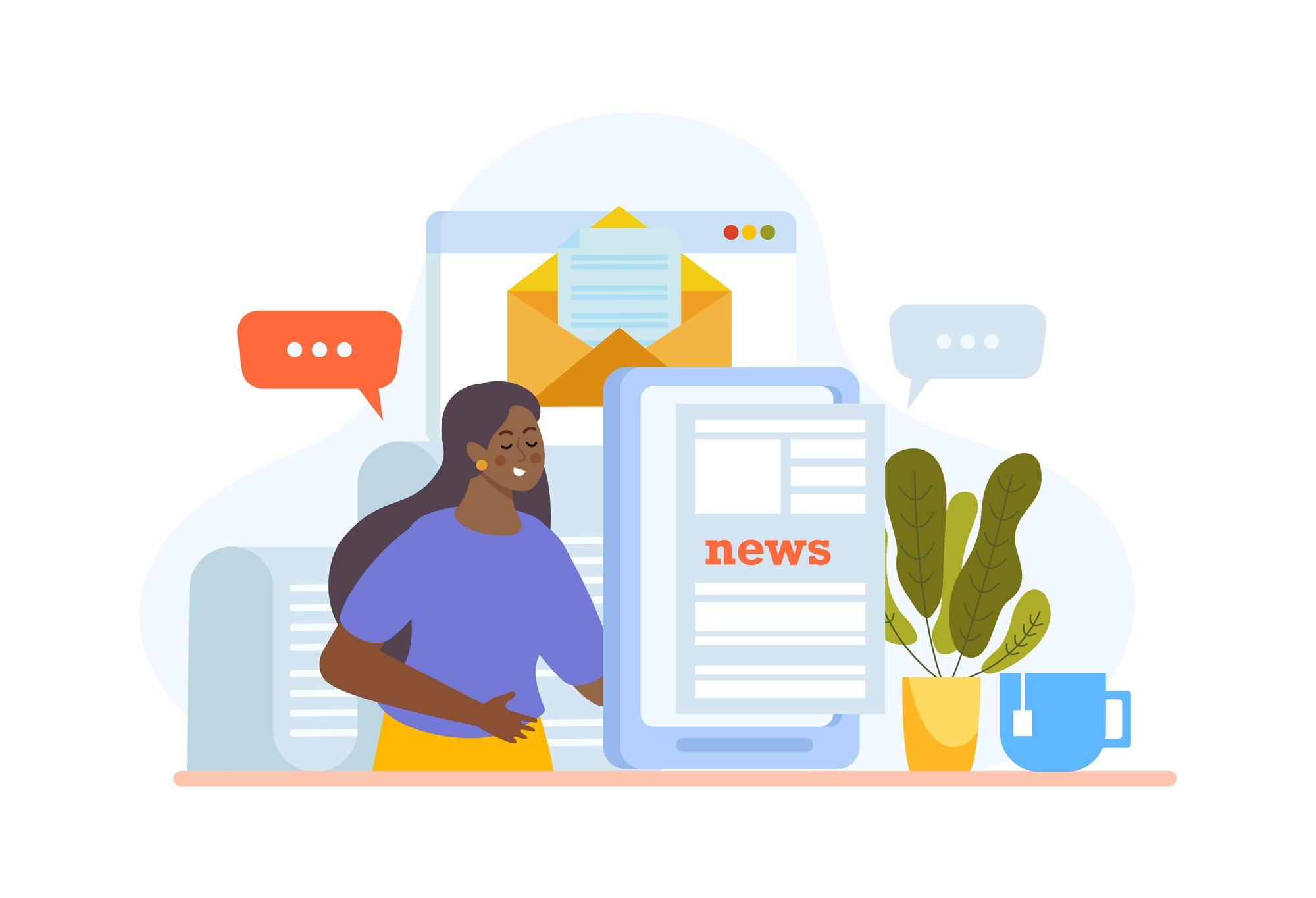
Why AI is Indispensable for 2025 Data Professionals
As data volumes explode and industries race to innovate, AI’s advantages are undeniable. Here’s why it is critical in 2025:
1. Automation Unleashes Human Potential
AI is predicted to automate most repetitive data work. DataRobot or AutoML in Google can build predictive models in just minutes—something that would take weeks to do before. This revolution allows experts to focus on high-impact projects, like developing ethical AI frameworks or creating data-driven innovations.
Example: AI automates a retail analyst’s inventory reports, freeing up 20 hours of a week. They now concentrate on developing hyper-personalized marketing plans based on AI-generated insights.
2. Predictive and Prescriptive Analytics Drive Smarter Decisions
Traditional analytics describes what happened; AI tells us what will happen. Retail giants leverage AI to predict demand, while medical systems forecast patient risks. Prescriptive AI takes it one step further by suggesting actionable tactics such as dynamic pricing or proactive maintenance.
Example: An AI algorithm examines traffic conditions and weather information to redirect delivery trucks in real-time, reducing logistics costs by 15%.
3. Unstructured Data Becomes an Untapped Goldmine
More than 80% of enterprise data is unstructured—social media posts, customer emails, or sensor logs. Conventional tools can’t handle this, but AI loves it. Natural Language Processing (NLP) reads sentiment from reviews, and computer vision identifies defects in manufacturing.
Example: A telecom operator employs NLP to process customer service calls, and they find common pain points and decrease the churn.
4. Real-Time Insights Define Competitive Edge
In finance or e-commerce, latencies cost millions. AI analyzes stream data in real-time, which enables real-time fraud detection (e.g., flagging suspicious transactions) or dynamic pricing (e.g., surge pricing for ride-sharing).
Example: AI-based fraud detection systems can help banks save $10 billion annually by detecting threats in milliseconds.
5. Scalability Keeps Pace with Big Data
AI scales with ease using cloud computing. Platforms such as AWS SageMaker or Azure ML can process petabytes of data, making them accessible for both startups and large corporations. Scalability guarantees that even small groups can use AI without significant infrastructure.
Example: A startup employs cloud-based AI software to process global supply chain data to find opportunities for savings without IT expenditure.
The Future of Data Professionals
AI isn’t displacing data professionals—it’s redefining their work. To succeed in 2025, professionals need to change:
- Upskill in AI and ML: Study Python, TensorFlow, or PyTorch to develop and decipher models.
- Master Data Storytelling: Bring complex AI information to life for stakeholders in terms of actionable strategy.
- Champion Ethics and Transparency: Make AI models fair, explainable, and regulatory-compliant.
- Collaborate with AI: Employ AI as a tool to augment—not replace—human intellect.
Example: A data scientist works with AI to analyze climate data, accelerating research into renewable energy sources.
Getting Ready for the AI-Powered Future
The introduction of AI makes lifelong learning essential. Organizations and institutions already prioritize interdisciplinary courses integrating data science, ethics, and domain expertise. For working professionals, staying up to date through certifications, workshops, and collaborations with industries will be essential.
Ethical Implications
As AI power increases, so does the need for responsibility. Professionals must address biases in training data, ensure privacy, and encourage transparent AI systems.
Data Analytics Related Articles | |
Conclusion
AI is forming the foundation for data analytics. Though traditional means hold niche significance, AI is the only viable choice with regard to speed, scalability, and prediction capabilities. Data professionals willing to leverage AI will spearhead innovation, harness new opportunities, and deliver a positive impact. Tomorrow is for changemakers—let the journey with AI begin.
The future of data is for those who are willing to adopt AI—not as an upgrade for choice, but as a strategic necessity. The age of AI-driven analytics is here — are you prepared to lead the revolution in 2025?
Previous post