Generative AI in Cybersecurity: A Beginner’s Guide to AI-Powered Threat Detection
- Date April 7, 2025
Generative AI in Cybersecurity: In this digital and interconnected age where safeguarding digital assets becomes critical, it is in view of this argument that generative AI is redefining the future of cybersecurity. This guide provides a thorough introduction to AI-enabled threat detection and investigates the intricate machinery of generative models in their contributions to enhancing defenses against elaborate cyber attacks. Theory and practice will educate the reader into a nuanced understanding of how cutting-edge AI methods will outsmart and extinguish progressively moving security challenges, leading to a more resilient digital infrastructure.
Must Read: How to Build a Successful Career in Cybersecurity
Generative AI in Cybersecurity
Generative AI refers to algorithms that generate new data instances by learning patterns from existing datasets. Contrary to traditional AI, which often deals with classification and prediction, generative AI can synthesize data, simulate attack scenarios, and model potential threat behaviors. These aspects are especially important for cybersecurity, as predicting new threats could mean the difference between a secure system and an all-out breach.
In the field of cybersecurity, generative AI models analyze extensive amounts of data from networks and logs from systems, combining them into realistic patterns of threat behavior. Cybersecurity evaluators can use understanding threats to thwart potential vulnerabilities before they can be exploited; learning from actual events allows these models to simulate potential breaching scenarios, further bolstering the organization’s proactive defense mechanism.
Best Cybersecurity Course
Looking to master AI in cybersecurity? EICT Academy, IIT Kanpur offers one of the best AI-powered cybersecurity courses designed for beginners and professionals alike. Learn real-world threat detection using Generative AI, hands-on tools, and expert guidance. Boost your career with a government-recognized program trusted by top tech recruiters.
Best Cybersecurity Course | |
Introduction to Cybercrime | |
Ethical Hacking for Beginners | |
Introduction to Cryptography | |
Introduction to Information Technology |
How Does AI-Powered Threat Detection Work?
Threat detection forms the very foundation of a good cybersecurity strategy. Old-fashioned threat detection methods are still ruled by resistance to parameters and signatures, which some emerging or sophisticated attacks may bypass. This is when AI-powered threat detection comes to put a stop to the game. In particular, generative AI variants bring several enhancements to the table.
Improved Detection Capabilities
Generative AI algorithms can create models that detect patterns in network traffic and user behavior so subtly as to raise red flags of an ongoing attack—namely, the streaming definition of what an incoming attack might be. This means an earlier diagnosis and healing, extracting the concept of the algorithm from the history of actual use. The technology can analyze real-time data and utilize them to indirectly improve its prediction power against emerging threats.
Real-Time Response and Predictive Analytics
As AI-powered threat detection systems retain the ability to analyze activity in real-time, they can notify unusual activity once again. One of the key advantages of these systems is that since such attacks evolve very rapidly, real-time notification becomes crucial in limiting the impact on business operations. In addition, predictive analytics built on generative AI may also help in forecasting attack vectors and reinforcing defenses in anticipation.
Also Read: Cyber Security Salary in 2025
Interested in E&ICT courses? Get a callback !
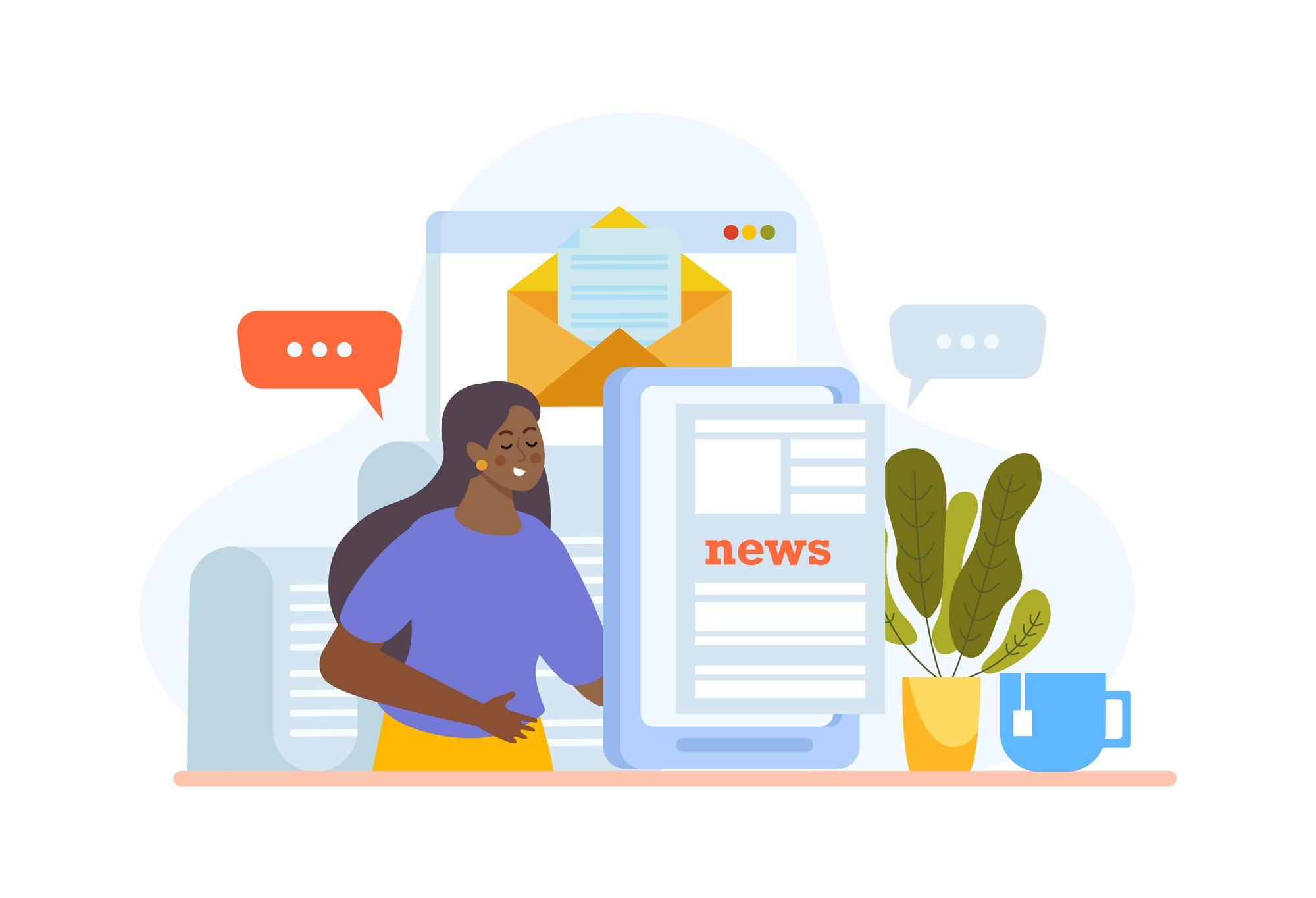
Key Advantages and Challenges
The incorporation of generative AI into cybersecurity has many advantages, as well as a few challenges.
Advantages
- Improved Accuracy: Generative AI reduces false positives by learning from large datasets, ensuring that those security alerts that are triggered are accurate and actionable.
- Analysis in Real-Time: Real-time data processing and analysis give organizations the advantage of immediate threat response.
- Predictive Capability: Generative models can assess the possibility of an occurring attack by depicting possibilities of Threats and applying preventive measures.
Challenges
- Data Quality: Everything regarding generative AI works well, depending upon the quality and diversity of available data. Uncertain data is going to undermine the performance of any generative AI system.
- Algorithm Complexity: Setting algorithms and maintaining operations with statistical methods are issues that require a high level of technical knowledge and constant vigilance.
- Adversarial Attacks: Whereas adversarial attacks attempt to influence AI models to mislead or evade an organization’s own security system, cyber attackers can either do so directly or in indirect ways.
A proper study of these benefits and challenges is relevant to both budding and veteran cybersecurity experts alike in finding their way through an ever-changing world of AI-enhanced security protocols.
Also Read: What is Post-Quantum Cryptography
Implementation Strategy for Beginners
A structured approach must be adopted for generative AI implementations in security for both organizations and individuals. The following are a few implementation strategies worth considering:
Also Read: Cyber Security vs Ethical Hacking
Step-To-Step Implementation
- Data Collection and Processing: Collect the complete dataset of network traffic, system logs, and historical attacks. Clean and preprocess this data to make it suitable for training the generative AI model.
- Model Training and Scenarios Simulations: Train different attack scenarios on generative AI models. Train these models based on historical data so that they can learn and predict threats to come.
- Continuous Monitoring and Updating: This process should be continuous and regularly updated to remain applicable against the evolving nature of attacks.
- Collaborations and Knowledge Sharing: Engage actively with academia and the research community to stay in touch with current developments in AI and cybersecurity. For further understanding, visit our Academics and Research pages for useful resources and educational programs.
Best Practices
- Set Clear Protocols: Clear data protection protocols, model training procedures, and model response actions must be developed to ensure that the integration of generative AI promotes and supports the entire system of cybersecurity.
- Train Well: Train cybersecurity personnel to use AI-driven tools. Engage them in periodic training sessions and certifications to improve their skill sets regarding new trends and technologies.
- Use Collaboration Platforms: Actualize AI model improvement by working together with scientists and technical analysts from the field. Check our Online Live Programs page for further seminars and workshops.
Cybersecurity Related Articles | |
Future Trends and Conclusion
The advancements in generative AI for cybersecurity are accelerating. By improving AI constructs and applying more efficient processes within organizations, the detection and neutralization of threats will be enhanced greatly. Advanced applications and seamless integrations with different types of security technology represent a practically unique experience in improving a security framework.
Generative AI establishes the basis for redefining detection with unprecedented capabilities in identifying and mitigating cyber risks. While there are challenges, its increased volume of precision and real-time response combined with predictive analytics make it necessary for modern cybersecurity practices. A structured implementation platform, combined with fresh views from agencies and research, would allow an efficient way to harness the power of this revolutionary tool and would lead to the construction of integrated systems that would ensure the safety of assets in cyberspace-their increasingly strategic function on a secure cyberspace.
Previous post